Node Graph Optimization Using Differentiable Proxies
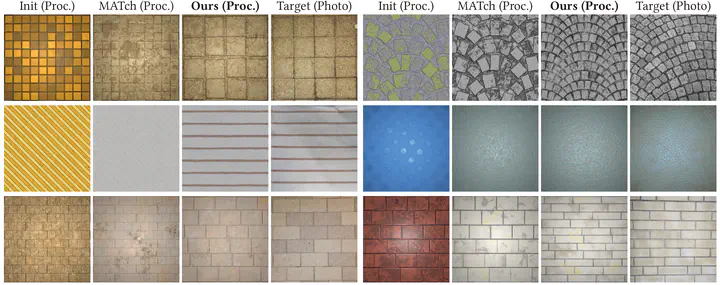
Abstract: Graph-based procedural materials are ubiquitous in content production industries. Procedural models allow the creation of photorealistic materials with parametric control for flexible editing of appearance. However, designing a specific material is a time-consuming process in terms of building a model and fine-tuning parameters. Previous work [Hu et al. 2022; Shi et al. 2020] introduced material graph optimization frameworks for matching target material samples. However, these previous methods were limited to optimizing differentiable functions in the graphs. In this paper, we propose a fully differentiable framework which enables end-to-end gradient based optimization of material graphs, even if some functions of the graph are non-differentiable. We leverage the Differentiable Proxy, a differentiable approximator of a non-differentiable black-box function. We use our framework to match structure and appearance of an output material to a target material, through a multi-stage differentiable optimization. Differentiable Proxies offer a more general optimization solution to material appearance matching than previous work.
Talk:
Results:
Click here to navigate our results.
Citation:
@inproceedings{hu2022diff,
author = {Hu, Yiwei and Guerrero, Paul and Hasan, Milos and Rushmeier, Holly and Deschaintre, Valentin},
title = {Node Graph Optimization Using Differentiable Proxies},
year = {2022},
isbn = {9781450393379},
publisher = {Association for Computing Machinery},
address = {New York, NY, USA},
url = {https://doi.org/10.1145/3528233.3530733},
doi = {10.1145/3528233.3530733},
booktitle = {ACM SIGGRAPH 2022 Conference Proceedings},
articleno = {5},
numpages = {9},
keywords = {inverse material modeling, procedural materials},
location = {Vancouver, BC, Canada},
series = {SIGGRAPH '22}
}